About sklearn.metrics.average_precision_score documentationHow to interpret the AUC score in this case?Where to find statistically relevant documentation of common Python packages?Irregular Precision-Recall CurveNeed a Work-around for OneHotEncoder Issue in SKLearn PreprocessingSci-kit learn function to select threshold for higher recall than precisionXGBoost: Quantifying Feature Importancessklearn nmf - question about its usemodel.predict in Keras, Python errorDifference between sklearn’s “log_loss” and “LogisticRegression”?Not sure if over-fitting
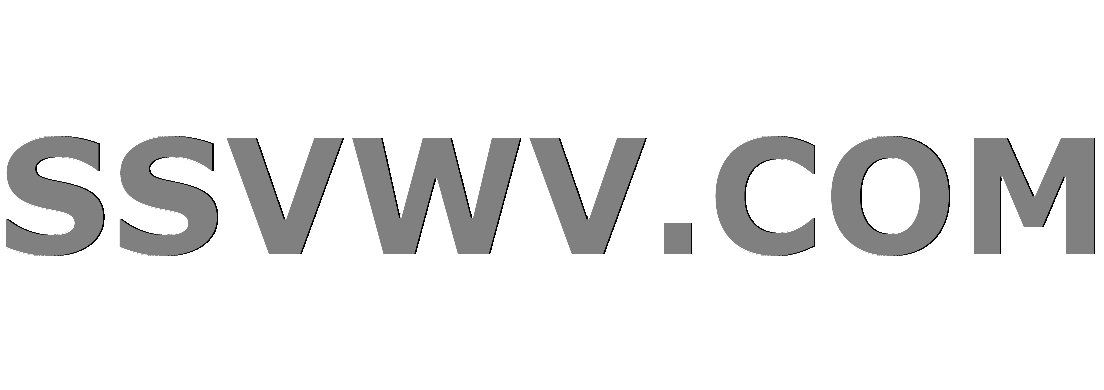
Multi tool use
About sklearn.metrics.average_precision_score documentation
What does this 'x' mean on the stem of the voice's note, above the notehead?
Very serious stuff - Salesforce bug enabled "Modify All"
Should I twist DC power and ground wires from a power supply?
How does the "reverse syntax" in Middle English work?
What does it mean for a program to be 32 or 64 bit?
Is there any official Lore on Keraptis the Wizard, apart from what is in White Plume Mountain?
Is being an extrovert a necessary condition to be a manager?
What should I wear to go and sign an employment contract?
"File type Zip archive (application/zip) is not supported" when opening a .pdf file
DISTINCT NULL return single NULL in SQL Server
Everytime I convert PDF to PNG it becomes more pixely. How can I fix it?
Bash Array of Word-Splitting Headaches
Does the Aboleth have expertise in history and perception?
Restraint classed as assault after suspecting unconsented photo
What were the "pills" that were added to solid waste in Apollo 7?
Good examples of "two is easy, three is hard" in computational sciences
Latin words remembered from high school 50 years ago
How can I prevent Bash expansion from passing files starting with "-" as argument?
Reference for electronegativities of different metal oxidation states
Why were early aviators' trousers flared at the thigh?
Why did Nick Fury not hesitate in blowing up the plane he thought was carrying a nuke?
Can 2 light bulbs of 120V in series be used on 230V AC?
Precedent for disabled Kings
About sklearn.metrics.average_precision_score documentation
How to interpret the AUC score in this case?Where to find statistically relevant documentation of common Python packages?Irregular Precision-Recall CurveNeed a Work-around for OneHotEncoder Issue in SKLearn PreprocessingSci-kit learn function to select threshold for higher recall than precisionXGBoost: Quantifying Feature Importancessklearn nmf - question about its usemodel.predict in Keras, Python errorDifference between sklearn’s “log_loss” and “LogisticRegression”?Not sure if over-fitting
$begingroup$
There is a example in sklearn.metrics.average_precision_score documentation.
import numpy as np
from sklearn.metrics import average_precision_score
y_true = np.array([0, 0, 1, 1])
y_scores = np.array([0.1, 0.4, 0.35, 0.8])
average_precision_score(y_true, y_scores)
0.83
But when I plot precision_recall_curve
precision, recall, _ = precision_recall_curve(y_true, y_scores)
plt.plot( recall,precision)
I got the picture:
why the area under the precision_recall_curve is not 0.83?
python scikit-learn scoring
$endgroup$
add a comment |
$begingroup$
There is a example in sklearn.metrics.average_precision_score documentation.
import numpy as np
from sklearn.metrics import average_precision_score
y_true = np.array([0, 0, 1, 1])
y_scores = np.array([0.1, 0.4, 0.35, 0.8])
average_precision_score(y_true, y_scores)
0.83
But when I plot precision_recall_curve
precision, recall, _ = precision_recall_curve(y_true, y_scores)
plt.plot( recall,precision)
I got the picture:
why the area under the precision_recall_curve is not 0.83?
python scikit-learn scoring
$endgroup$
add a comment |
$begingroup$
There is a example in sklearn.metrics.average_precision_score documentation.
import numpy as np
from sklearn.metrics import average_precision_score
y_true = np.array([0, 0, 1, 1])
y_scores = np.array([0.1, 0.4, 0.35, 0.8])
average_precision_score(y_true, y_scores)
0.83
But when I plot precision_recall_curve
precision, recall, _ = precision_recall_curve(y_true, y_scores)
plt.plot( recall,precision)
I got the picture:
why the area under the precision_recall_curve is not 0.83?
python scikit-learn scoring
$endgroup$
There is a example in sklearn.metrics.average_precision_score documentation.
import numpy as np
from sklearn.metrics import average_precision_score
y_true = np.array([0, 0, 1, 1])
y_scores = np.array([0.1, 0.4, 0.35, 0.8])
average_precision_score(y_true, y_scores)
0.83
But when I plot precision_recall_curve
precision, recall, _ = precision_recall_curve(y_true, y_scores)
plt.plot( recall,precision)
I got the picture:
why the area under the precision_recall_curve is not 0.83?
python scikit-learn scoring
python scikit-learn scoring
edited 2 hours ago
Ben Reiniger
664214
664214
asked 6 hours ago


disney82231disney82231
352
352
add a comment |
add a comment |
1 Answer
1
active
oldest
votes
$begingroup$
According to the documentation, the value is not exactly the area under curve, it is
$$textAP = sum_n(R_n - R_n-1)P_n.$$
which is a rectangular approximation.
For your specific example, it is calculated as
$$beginalign*
textAP & = overbrace(0.5 - 0.0)times1.0^(R_2 - R_1)P_2 + overbrace(0.5 - 0.5)times 0.5^(R_3 - R_2)P_3 + overbrace(1.0 - 0.5) times0.66^(R_4 - R_3)P_4 \
&= 0.5 + 0.00+ 0.33 = 0.83
endalign*$$
which is the area under the red curve as illustrated below:
$endgroup$
3
$begingroup$
Beat me to it; in particular, the documentation states "This implementation is not interpolated and is different from computing the area under the precision-recall curve with the trapezoidal rule, which uses linear interpolation and can be too optimistic."
$endgroup$
– Ben Reiniger
2 hours ago
add a comment |
Your Answer
StackExchange.ready(function()
var channelOptions =
tags: "".split(" "),
id: "557"
;
initTagRenderer("".split(" "), "".split(" "), channelOptions);
StackExchange.using("externalEditor", function()
// Have to fire editor after snippets, if snippets enabled
if (StackExchange.settings.snippets.snippetsEnabled)
StackExchange.using("snippets", function()
createEditor();
);
else
createEditor();
);
function createEditor()
StackExchange.prepareEditor(
heartbeatType: 'answer',
autoActivateHeartbeat: false,
convertImagesToLinks: false,
noModals: true,
showLowRepImageUploadWarning: true,
reputationToPostImages: null,
bindNavPrevention: true,
postfix: "",
imageUploader:
brandingHtml: "Powered by u003ca class="icon-imgur-white" href="https://imgur.com/"u003eu003c/au003e",
contentPolicyHtml: "User contributions licensed under u003ca href="https://creativecommons.org/licenses/by-sa/3.0/"u003ecc by-sa 3.0 with attribution requiredu003c/au003e u003ca href="https://stackoverflow.com/legal/content-policy"u003e(content policy)u003c/au003e",
allowUrls: true
,
onDemand: true,
discardSelector: ".discard-answer"
,immediatelyShowMarkdownHelp:true
);
);
Sign up or log in
StackExchange.ready(function ()
StackExchange.helpers.onClickDraftSave('#login-link');
);
Sign up using Google
Sign up using Facebook
Sign up using Email and Password
Post as a guest
Required, but never shown
StackExchange.ready(
function ()
StackExchange.openid.initPostLogin('.new-post-login', 'https%3a%2f%2fdatascience.stackexchange.com%2fquestions%2f52130%2fabout-sklearn-metrics-average-precision-score-documentation%23new-answer', 'question_page');
);
Post as a guest
Required, but never shown
1 Answer
1
active
oldest
votes
1 Answer
1
active
oldest
votes
active
oldest
votes
active
oldest
votes
$begingroup$
According to the documentation, the value is not exactly the area under curve, it is
$$textAP = sum_n(R_n - R_n-1)P_n.$$
which is a rectangular approximation.
For your specific example, it is calculated as
$$beginalign*
textAP & = overbrace(0.5 - 0.0)times1.0^(R_2 - R_1)P_2 + overbrace(0.5 - 0.5)times 0.5^(R_3 - R_2)P_3 + overbrace(1.0 - 0.5) times0.66^(R_4 - R_3)P_4 \
&= 0.5 + 0.00+ 0.33 = 0.83
endalign*$$
which is the area under the red curve as illustrated below:
$endgroup$
3
$begingroup$
Beat me to it; in particular, the documentation states "This implementation is not interpolated and is different from computing the area under the precision-recall curve with the trapezoidal rule, which uses linear interpolation and can be too optimistic."
$endgroup$
– Ben Reiniger
2 hours ago
add a comment |
$begingroup$
According to the documentation, the value is not exactly the area under curve, it is
$$textAP = sum_n(R_n - R_n-1)P_n.$$
which is a rectangular approximation.
For your specific example, it is calculated as
$$beginalign*
textAP & = overbrace(0.5 - 0.0)times1.0^(R_2 - R_1)P_2 + overbrace(0.5 - 0.5)times 0.5^(R_3 - R_2)P_3 + overbrace(1.0 - 0.5) times0.66^(R_4 - R_3)P_4 \
&= 0.5 + 0.00+ 0.33 = 0.83
endalign*$$
which is the area under the red curve as illustrated below:
$endgroup$
3
$begingroup$
Beat me to it; in particular, the documentation states "This implementation is not interpolated and is different from computing the area under the precision-recall curve with the trapezoidal rule, which uses linear interpolation and can be too optimistic."
$endgroup$
– Ben Reiniger
2 hours ago
add a comment |
$begingroup$
According to the documentation, the value is not exactly the area under curve, it is
$$textAP = sum_n(R_n - R_n-1)P_n.$$
which is a rectangular approximation.
For your specific example, it is calculated as
$$beginalign*
textAP & = overbrace(0.5 - 0.0)times1.0^(R_2 - R_1)P_2 + overbrace(0.5 - 0.5)times 0.5^(R_3 - R_2)P_3 + overbrace(1.0 - 0.5) times0.66^(R_4 - R_3)P_4 \
&= 0.5 + 0.00+ 0.33 = 0.83
endalign*$$
which is the area under the red curve as illustrated below:
$endgroup$
According to the documentation, the value is not exactly the area under curve, it is
$$textAP = sum_n(R_n - R_n-1)P_n.$$
which is a rectangular approximation.
For your specific example, it is calculated as
$$beginalign*
textAP & = overbrace(0.5 - 0.0)times1.0^(R_2 - R_1)P_2 + overbrace(0.5 - 0.5)times 0.5^(R_3 - R_2)P_3 + overbrace(1.0 - 0.5) times0.66^(R_4 - R_3)P_4 \
&= 0.5 + 0.00+ 0.33 = 0.83
endalign*$$
which is the area under the red curve as illustrated below:
answered 2 hours ago
EsmailianEsmailian
4,519422
4,519422
3
$begingroup$
Beat me to it; in particular, the documentation states "This implementation is not interpolated and is different from computing the area under the precision-recall curve with the trapezoidal rule, which uses linear interpolation and can be too optimistic."
$endgroup$
– Ben Reiniger
2 hours ago
add a comment |
3
$begingroup$
Beat me to it; in particular, the documentation states "This implementation is not interpolated and is different from computing the area under the precision-recall curve with the trapezoidal rule, which uses linear interpolation and can be too optimistic."
$endgroup$
– Ben Reiniger
2 hours ago
3
3
$begingroup$
Beat me to it; in particular, the documentation states "This implementation is not interpolated and is different from computing the area under the precision-recall curve with the trapezoidal rule, which uses linear interpolation and can be too optimistic."
$endgroup$
– Ben Reiniger
2 hours ago
$begingroup$
Beat me to it; in particular, the documentation states "This implementation is not interpolated and is different from computing the area under the precision-recall curve with the trapezoidal rule, which uses linear interpolation and can be too optimistic."
$endgroup$
– Ben Reiniger
2 hours ago
add a comment |
Thanks for contributing an answer to Data Science Stack Exchange!
- Please be sure to answer the question. Provide details and share your research!
But avoid …
- Asking for help, clarification, or responding to other answers.
- Making statements based on opinion; back them up with references or personal experience.
Use MathJax to format equations. MathJax reference.
To learn more, see our tips on writing great answers.
Sign up or log in
StackExchange.ready(function ()
StackExchange.helpers.onClickDraftSave('#login-link');
);
Sign up using Google
Sign up using Facebook
Sign up using Email and Password
Post as a guest
Required, but never shown
StackExchange.ready(
function ()
StackExchange.openid.initPostLogin('.new-post-login', 'https%3a%2f%2fdatascience.stackexchange.com%2fquestions%2f52130%2fabout-sklearn-metrics-average-precision-score-documentation%23new-answer', 'question_page');
);
Post as a guest
Required, but never shown
Sign up or log in
StackExchange.ready(function ()
StackExchange.helpers.onClickDraftSave('#login-link');
);
Sign up using Google
Sign up using Facebook
Sign up using Email and Password
Post as a guest
Required, but never shown
Sign up or log in
StackExchange.ready(function ()
StackExchange.helpers.onClickDraftSave('#login-link');
);
Sign up using Google
Sign up using Facebook
Sign up using Email and Password
Post as a guest
Required, but never shown
Sign up or log in
StackExchange.ready(function ()
StackExchange.helpers.onClickDraftSave('#login-link');
);
Sign up using Google
Sign up using Facebook
Sign up using Email and Password
Sign up using Google
Sign up using Facebook
Sign up using Email and Password
Post as a guest
Required, but never shown
Required, but never shown
Required, but never shown
Required, but never shown
Required, but never shown
Required, but never shown
Required, but never shown
Required, but never shown
Required, but never shown
ZG8xxdyEF7lv58C3gxUz6,P7,PZYRNot OEgx rBg9IfMXn8KEP,CAh C4VDz0sFDTO9vbabd1I2 Io8iCTIV,sY3LW2BZ P9hv9VFJ